Advanced Networking for AI and ML: Scaling to Meet Tomorrow’s Demand
Traditional data center architectures cannot support the scale and complexity of modern AI workloads. To keep pace with unprecedented training and inference compute demands, the industry requires innovative physical layer solutions, including energy-efficient, high-performing hardware and advanced cooling techniques.
AI Data Center Energy Consumption and Cooling Solutions
AI data centers use powerful GPUs to create large language models (LLMs) through training and inference. Training adjusts the model’s parameters for better pattern recognition, while inference uses the trained model to make predictions. Due to the complexity and high-power consumption of building LLMs, AI data centers use much more energy than traditional data centers. For example, while standard enterprise rack power density increased from about 5 kW to 8-10 kW between 2017 and 2022, modern AI racks start at around 15-20 kW, with high-performance AI racks reaching 30-50 kW (some exceptional cases reach around 200 kW).
The immense power consumption also generates substantial heat, necessitating advanced cooling solutions. Traditional air-cooling methods prove insufficient for high-density AI data center environments. As a result, advanced thermal management techniques have become more prevalent, including direct-to-chip cooling – managing heat loads exceeding 100 kW per rack – and immersion cooling. Although immersion cooling offers significant potential, the method faces environmental and equipment compatibility challenges. Despite these issues, immersion cooling effectively cools high-temperature AI data centers and may yet see wider adoption.
Physical Space and Network Topologies
AI data centers must expand to accommodate the modern, specialized hardware and cooling systems required for optimal performance. Future projects, such as Microsoft and OpenAI’s Stargate facility, indicate power requirements of several gigawatts, with a footprint potentially spanning hundreds of acres. This trend in growing AI data center footprints underscores the need for scalable, efficient network topologies.
Network topology choice is central to efficient data flow. Two such examples include Clos and torus topologies. Clos topologies provide non-blocking, high-bandwidth connectivity, minimizing congestion for effective data transfer, while torus topologies offer low-latency communication between nodes, enhancing performance and scalability. Many other topologies offer specific networking solutions. Operators often deploy hybrid topologies to support modern AI clusters.
The Backend and Frontend Networks
To optimize data flow and processing efficiency, AI data centers require a distinct, purpose-designed frontend network (FENW) and backend network (BENW). The FENW connects every node to orchestrate traffic and telemetry. Set up correctly, the FENW facilitates seamless data access, integration, and efficient communication across the network. The BENW connects each accelerator, enabling components to share model update information during training. Efficient BENWs create low-latency, high-speed memory-sharing environments.
Scalability and Fault Tolerance
As detailed in our recent white paper, a well-architected Clos network can support thousands to hundreds of thousands of endpoints. Operators achieve this extensive connectivity by incorporating additional leaf and spine switches into the network. Clos architectures enhance fault tolerance and redundancy, ensuring continuous network performance even if individual components fail.
Let’s dig a little deeper. For instance, a Clos topology designed to support 131,072 endpoints would require 5,120 switches and 131,072 optical links. The approximate power consumption for such a network would equal 9,646 kW, highlighting the significant energy demands of large-scale AI workloads.
Innovative Connectivity Solutions
The high-density connections per rack in AI/Machine Learning (ML) servers and switches necessitate advanced connector solutions. Multi-fiber MPO connectors and next-generation very high-density connectors, such as MMC and SN-MT (next-generation form factors for 400G and 800G connectivity), are emerging in the context of the broader data center market, with growing wider adoption in hyperscale environments. These connectors support high-speed, reliable connectivity, essential for maintaining data integrity and optimizing performance.
Due to factors such as high bandwidth and resistance to electromagnetic interference, optical fiber cables are the preferred choice for AI data centers. Proper cable management is crucial for expedited and simplified troubleshooting.
The Future of AI Data Centers
The AI/ML landscape is evolving. To remain competitive, advanced AI data centers must invest in high-performance, energy-efficient AI hardware, optical fiber solutions, and advanced cooling methods.
Conclusion
The growing demand for AI and ML technologies has vastly transformed modern data center infrastructures. While adjustments to keep up with demand may have at first been seen as a technical necessity, AI’s unstoppable momentum arguably creates a strategic imperative to innovate today or fall by the wayside tomorrow.
By embracing advanced networking solutions and innovative physical layer technologies, data center operators can confidently and quickly achieve the performance, scalability, and efficiency required to drive the next generation of AI innovations. One thing is clear: the shift towards newer AI technologies and advanced AI data center networking represents a pivotal opportunity for industry leaders to invest in future operations and remain at the forefront of the technological advancements customers demand.
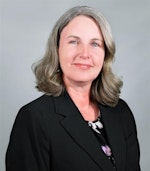
Manja Thessin
Manja Thessin RCDD/RTPM serves as Enterprise Market Manager for AFL, leading strategic planning and market analysis initiatives. Manja has more than 20 years of ICT experience in the field, design-and-engineering, and project management. She has managed complex initiatives in Data Center, Education, Industrial/Manufacturing and Healthcare. Manja earned a master’s certificate from Michigan State University in Strategic Leadership and holds RCDD and RTPM certifications from BICSI.
Guided by customer-first thinking, AFL has experienced remarkable growth coupled with outstanding market recognition. As a major player in the optical fiber hyperscale space, AFL contributes consistent industry messaging, offering readers the opportunity to stay current with emerging hyperscale technologies and the evolution of the AI data center landscape. AFL’s latest white paper, titled Advanced Networks for Artificial Intelligence and Machine Learning Computing: Scaling Fiber Networks to Meet Tomorrow’s Data Center Demands, enhances reader perceptions of networking for complex AI workloads.